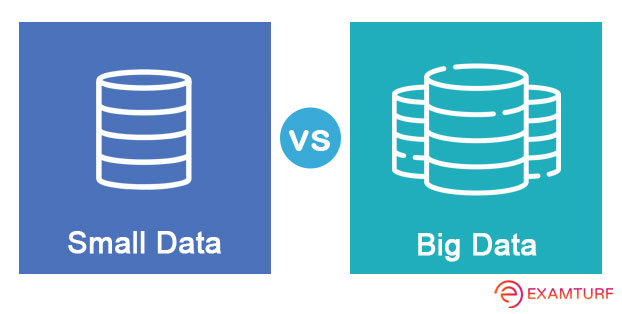
Definition of Small Data vs Big Data
Small data vs big data differ from each other. Small data with current decision-impacting frequency is referred to as small data and it contains a small volume of data so humans easily understood. The big data phrase has gained a lot of popularity in recent years. It is complex for humans to process large volumes of data. Big data processing is not quite easy as compared to small data.
Table of contents
Difference Between Small Data vs Big Data
In terms of, small data it doesn’t have as much of an influence as big data. It affects judgments that are made now and in the near future more than anything else. Big data refers to the enormous amounts of data produced digitally, such as online data produced by social websites, streaming, and many more. Big data is complicated to be analyzed by techniques of traditional data processing also referred to as big data.
The volume of data is nothing but data available for processing. Large information is needed for big data, but for small data, we need small information. Small data contains limited risks, whereas big data contains high risks. We can keep the small data-limited period of time, but big data contains large space to store it as per requirement.
What is Small Data?
Small Data is similarly useful for decision-making, but it only seeks to have a small, temporary impact on the business. Data that is small enough for humans to understand in terms of both format and volume is referred to as small data. The volume of data signifies how much it needs to be processed. Additionally, the amount is significantly lower for little data, which could imply more accurate, bite-sized metrics.
The phrase “small data” contrasts with the phrase “big data,” refers to material that is too big and complex to be examined and processed using conventional data-processing methods. Small data, in contrast to Big Data, enter at a steady and regulated rate for processing, and they accumulate at a relatively modest rate as well, making them simple to analyze and readily available.
What is Big Data?
Big Data is the term for enormous, complicated data sets that are too large and complex to be evaluated and processed using methods of conventional data processing. Big Data is the term used to describe the vast quantities of information created in the digital era, which includes all of the Web data produced by social networking sites, streaming services, and email and website traffic.
According to estimates, the rise of the Internet of Things and the rising number of digital devices result in the creation of roughly 2.5 quintillion bytes every day. Additionally, social networking sites produce a significant amount of data every minute in the form of photographs, videos, and graphics.
Head to Head Comparison Between Small Data vs Big Data (Infographics)
Below are the top 12 differences between Small Data and Big Data:
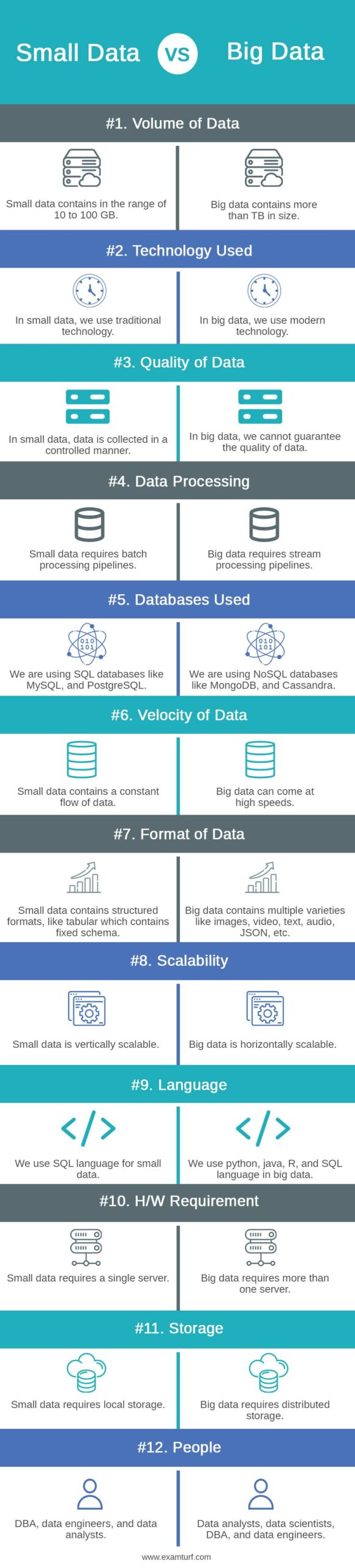
Key Differences Between Small Data and Big Data
Let us look at the key differences between Small Data and Big Data:
- Small data is contained in a structured format, whereas big data does not come in a structured format, it is a combination of structured and unstructured data. Big data comes from social media, real-time analytics, and many more sources.
- We can refer to small data as data is tiny in format and it will comprehend humans in terms of format and volume. Big data refers to data that is complex and is processed by using techniques of data processing.
- Examining the velocity of data generated in real-time by user clicks is the best technique to calculate big data velocity. Small data, deals with one particular sort of data, therefore data buildup in this context is relatively moderate and data flow is continuous and controlled.
- Big Data comes in forms, including unstructured, semi-structured, and structured. Emails, messages, and document data are called big data. The small data comes from transaction systems.
- We can store big data in distributed storage like external file systems or Hadoop. We can store small data in a single machine or local computer.
Small Data Requirement
Small Data is a method that emphasizes the caliber rather than the quantity of information gathered. While using small data we need to check below things are as follows.
- While using small data in our business we need to make sure that our application does not generate more data.
- At the time of dealing with small data, we need to implement good infrastructure where we can safely keep our data.
- We need to do augmentation on data, techniques of augmentation allows us to produce a training model.
- By using small data we can generate synthetic data, this data is useful for analysis.
- At the time of using small data, we need to split our datasets and we need to train and test the same.
- We need to use transfer learning techniques in our dataset.
Big Data Requirement
To make the information relevant and useful for making sound business judgments, big data must extensively investigate the entire subject. Below are the requirements of big data as follows.
- The first requirement of big data is data processing, this feature includes the organization and collection of raw data.
- The second requirement of big data is predictive applications, in that we need to identify the works of management.
- We need to use analytics tools for offering flexibility, those tools contain multiple packages.
- We need to do risk analysis studies of a certain activity.
- The analytical tools of big data contain the module name decision management which implements the process of business.
- We need to use text analytics which examines text which was written.
Comparison Table of Small Data vs Big Data
The table below summarizes the comparisons between Small Data vs Big Data:
Points | Small Data | Big Data |
Volume of data | Small data contains in the range of 10 to 100 GB. | Big data contains more than TB in size. |
Technology used | In small data, we use traditional technology. | In big data, we use modern technology. |
Quality of data | In small data, data is collected in a controlled manner. | In big data, we cannot guarantee the quality of data. |
Data processing | Small data requires batch processing pipelines. | Big data requires stream processing pipelines. |
Databases used | We are using SQL databases like MySQL, and PostgreSQL. | We are using NoSQL databases like MongoDB, and Cassandra. |
Velocity of data | Small data contains a constant flow of data. | Big data can come at high speeds. |
Format of data | Small data contains structured formats, like tabular which contains fixed schema. | Big data contains multiple varieties like images, video, text, audio, JSON, etc. |
Scalability | Small data is vertically scalable. | Big data is horizontally scalable. |
Language | We use SQL language for small data. | We use python, java, R, and SQL language in big data. |
H/W Requirement | Small data requires a single server. | Big data requires more than one server. |
Storage | Small data requires local storage. | Big data requires distributed storage. |
People | DBA, data engineers, and data analysts. | Data analysts, data scientists, DBA, and data engineers. |
Purpose of Small Data
Small data is easy to manage also it comes in structured and tabular formats. Small data that have an immediate impact on decisions are known as small data. Any activity that is now taking place and whose data may be compiled inside an Excel. Making decisions with small amounts of data is also helpful.
Small data consists of distinct and focused dataset features that can be utilized to evaluate present circumstances. Small Data also refers to the unique datasets discovered after sifting through enormous amounts of data. To store small data we are using RDBMS databases like MySQL, PostgreSQL, MSSQL, and Oracle.
Purpose of Big Data
Big Data is beneficial to company owners who must make critical expansion decisions. Using Big Data Analytics, which has a good effect on business, we depend on a team of experts to extract usable data. Businesses can make critical decisions and proceed in the right direction thanks to the insights gained by a Big Data expert.
Big Data tools are widely accessible in the market, simplifying the work of analysts and promoting business growth. Big data is useful when managing vast amounts of data. We are managing big data by using a database like MongoDB, and Cassandra.
Conclusion
The volume of data is nothing but data available for processing. Large information is needed for big data, but for small data, we need small information. Small data contains limited risks, whereas big data contains high risks. Small data with current decision-impacting frequency is referred to as small data and it contains a small volume of data so humans easily understood it.
Recommended Articles
This is a guide to Small Data vs Big Data. Here we discuss Small Data vs Big Data key differences with infographics and a comparison table in detail. You can also go through our other suggested articles to learn more –
Are you preparing for the entrance exam ?
Join our Data Science test series to get more practice in your preparation
View More