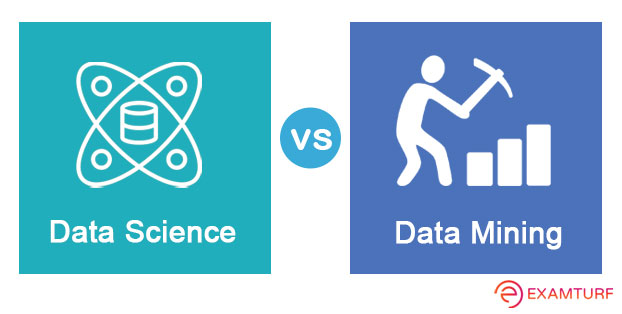
Definition of Data Science vs Data Mining
We know that data science is a field that contains the processing of data with huge amounts and making the prediction of results as well as prescriptive models. A prescriptive model is nothing but the dagging or capturing of data and analysis means data validation. On the other hand, data mining is a technique that extracts the data from a large amount of data set and makes a prediction such as a review of the product.
Difference Between Data Science vs Data Mining
Data Science: Data science is a field or domain that uses a lot of data to build predictive, prescriptive, and predictive analytical models and includes and involves working with a lot of data. Digging, capturing, analyzing (validating the model), and making use of the data (deploying the best model) are all involved.
Data and computing are at odds here. It is a combination of statistics, business, and computer science.
Data Mining: The process of extracting crucial information and knowledge from a vast data set or libraries is known as data mining. By carefully extracting, reviewing, and processing the vast amount of data, it discovers patterns and co-relations that may be crucial to the business and provides insight. Let’s consider a gold example: it is collected from sand and rocks.
What is Data Science?
Data science combines technology, algorithm development, and data interference to study, analyze, and discover novel approaches to complex problems. Analyzing data and finding inventive ways to drive business growth are the primary focuses of data science. To put it in another way, we had a lot of data with us, but we couldn’t figure out anything from it. Data Science was born out of the need to better understand and use data to make decisions. The architecture is shown in the screenshot below.
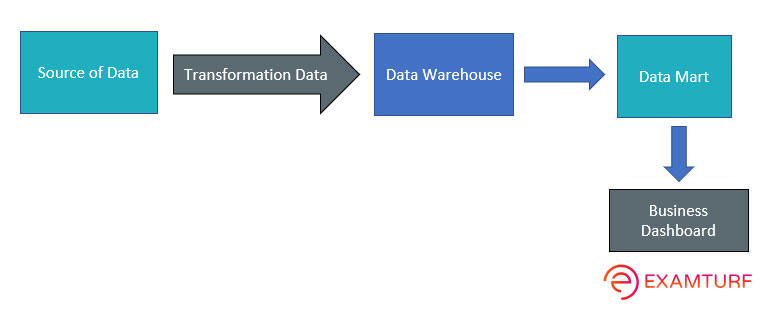
What is Data Mining?
The extraction of useful data, patterns, and trends from raw data is known as data mining. Data mining divides the data and evaluates the likelihood of future events using sophisticated mathematical algorithms. Data mining processes include text mining, web mining, audio and video mining, pictorial data mining, and social network data mining, among other types of services. Information mining is finished through straightforward or high-level programming. Knowledge Discovery in Data (KDD) is the name given to data mining. The architecture is shown in the screenshot below.
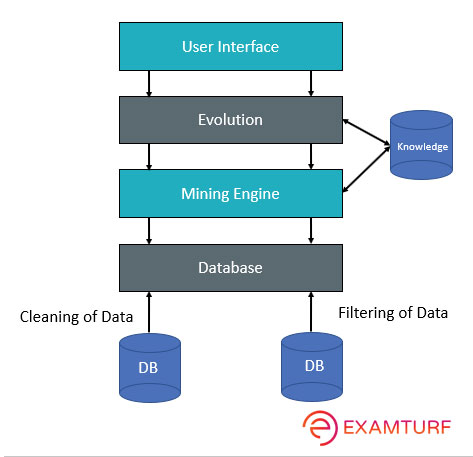
Head to Head Comparison Between Data Science vs Data Mining (Infographics)
Below are the top 6 differences between Data Science and Data Mining:
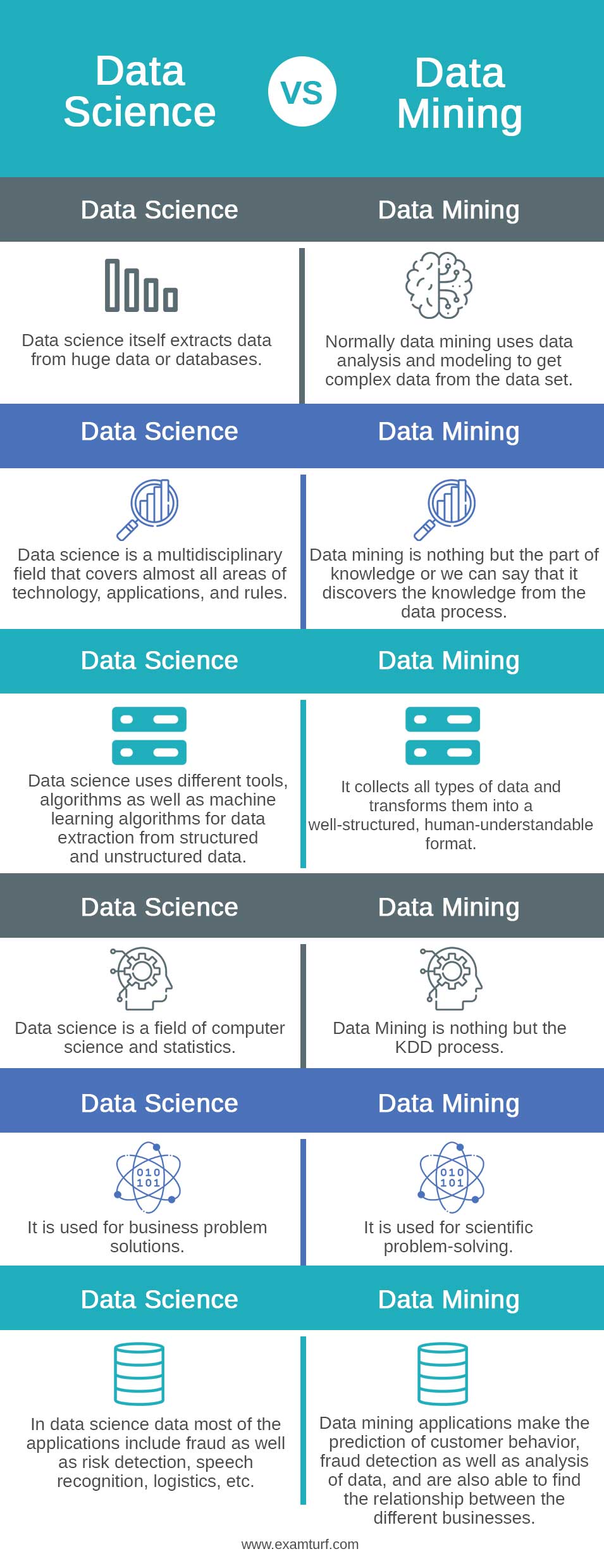
Key Differences
Let us look at the key differences between Data Science and Data Mining:
- Data collection, data analysis, and insight extraction all fall under the umbrella of data science. Data mining is using a dataset to find hidden patterns before using the information to find useful information.
- Statistics, the social sciences, data visualization, natural language processing, and data mining make up the multidisciplinary field of data science. A subset of data science is data mining.
- No matter whether the data is structured, semi-structured, or unstructured, data science relies on it all. Most of the time, only structured data is used in data mining.
- While data mining only became popular in the past days, data science has been around since the 1960s.
- Data mining is more concerned with the actual process, whereas data science focuses on the science of data.
Data Science Requirement
The following Technical Skills are required:
- Statistical Analysis.
- Processing or Data Mining.
- Development knowledge.
- Knowledge of different analytical tools such as SAS
- Able to work with unstructured data sets.
The following Non-Technical Skills are required
- Strong business knowledge.
- Communication skills must be required.
- Data handling knowledge.
Data Mining Requirements
Required Technical skills
- Able to handle huge amounts of data.
- Visualization of data is one of the key points.
- Statistical Analysis.
- Development knowledge
- Manipulation of data is also required.
- Some calculus or we can say that Linear Algebra knowledge.
Required Non-Technical skills
- Strong business knowledge.
- Communication skills must be required.
- Strong Intellectual skills.
Comparison Table of Data Science vs Data Mining
The table below summarizes the comparisons between Data Science and Data Mining:
Data Mining | Data Science |
Normally data mining uses data analysis and modeling to get complex data from the data set. | Data science itself extracts data from huge data or databases. |
Data mining is nothing but the part of knowledge or we can say that it discovers the knowledge from the data process. | Data science is a multidisciplinary field that covers almost all areas of technology, applications, and rules. |
It collects all types of data and transforms them into a well-structured, human-understandable format. | Data science uses different tools, algorithms as well as machine learning algorithms for data extraction from structured and unstructured data. |
Data Mining is nothing but the KDD process. | Data science is a field of computer science and statistics. |
It is used for scientific problem-solving. | It is used for business problem solutions. |
Data mining applications make the prediction of customer behavior, fraud detection as well as analysis of data, and are also able to find the relationship between the different businesses. | In data science data most of the applications include fraud as well as risk detection, speech recognition, logistics, etc. |
Purpose of Data Science
- With the help of data science, we can easily implement artificial intelligence, machine, and deep learning applications.
- Decision-making is easy because here we do some mathematical calculations which means it makes sense of the data.
- We can easily implement an automated recruitment process as well as it provides a better solution to the business.
- One of the most important advantages is that it reduces the risks.
Purpose of Data Mining
- It helps to collect the reliable or required data set.
- Compared to other applications it is cost-effective and efficient.
- It provides the solution to finance and banking to determine the credit score.
- By using data mining we can easily determine the different groups of customers.
- One of the most important advantages is that it helps us to increase the revenue of the company.
Conclusion
From this article, we understand Data Science vs Data mining. It provides the basic idea and implementation of Data Science vs Data mining and we also see the representation of Data science vs Data mining. In the end, we get an idea about the uses of Data science vs Data mining.
Recommended Articles
This is a guide to Data Science vs Data Mining. Here we discuss Data Science vs Data Mining key differences with infographics and a comparison table in detail. You can also go through our other suggested articles to learn more –
- Data Science vs Data Engineering
- Data Science vs Computer Science
- Data Science vs Machine Learning
- Big Data vs Data Science
Are you preparing for the entrance exam ?
Join our Data Science test series to get more practice in your preparation
View More